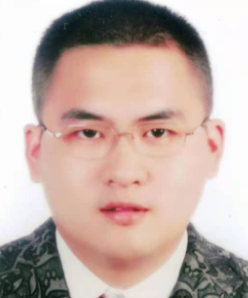
姚帅寓
- 教师英文名称: Alvin Yao
- 教师拼音名称: Yao Shuaiyu
- 电子邮箱:
- 学历: 博士研究生毕业
- 主要任职: 助理研究员
- 毕业院校: 曼彻斯特大学
- 学科:系统工程
开通时间:..
最后更新时间:..
点击次数:
影响因子:2.0
DOI码:10.3233/JIFS-201833
发表刊物:Journal of Intelligent & Fuzzy Systems
刊物所在地:NETHERLANDS
关键字:Probabilistic modeling; Interpretable inference and classification; Maximum likelihood evidential reasoning (MAKER) framework; Belief rule-base; Machine learning
摘要:In this paper, we propose a new probabilistic modeling approach for interpretable inference and classification using the maximum likelihood evidential reasoning (MAKER) framework. This approach integrates statistical analysis, hybrid evidence combination and belief rule-based (BRB) inference, and machine learning. Statistical analysis is used to acquire evidence from data. The BRB inference is applied to analyze the relationship between system inputs and outputs. An interdependence index is used to quantify the interdependence between input variables. An adapted genetic algorithm is applied to train the models. The model established by the approach features a unique strong interpretability, which is reflected in three aspects: (1) interpretable evidence acquisition, (2) interpretable inference mechanism, and (3) interpretable parameters determination. The MAKER-based model is shown to be a competitive classifier for the Banana, Haberman’s survival, and Iris data set, and generally performs better than other interpretable classifiers, e.g., complex tree, logistic regression, and naive Bayes.
论文类型:期刊论文
学科门类:工学
文献类型:J
卷号:40
期号:3
页面范围:5101-5117
ISSN号:1064-1246
是否译文:否
发表时间:2021-03-02
收录刊物:SCI(E)