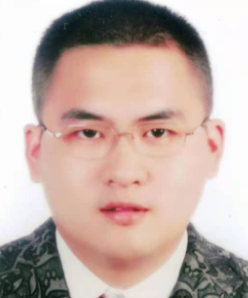
姚帅寓
- 教师英文名称: Alvin Yao
- 教师拼音名称: Yao Shuaiyu
- 电子邮箱:
- 学历: 博士研究生毕业
- 主要任职: 助理研究员
- 毕业院校: 曼彻斯特大学
- 学科:系统工程
开通时间:..
最后更新时间:..
点击次数:
DOI码:10.1109/ICNSC55942.2022.10004090
发表刊物:2022 IEEE International Conference on Networking, Sensing and Control (ICNSC)
关键字:Interaction Graph; Graph Convolutional Network; Deep Learning; Recommendation Systems
摘要:Graph convolutional neural network is a deep learning model for graph-structured data. It has become a popular approach for recommendation system research due to its powerful feature extraction and characterization learning capabilities. As for rating prediction in recommendation systems, most existing models based on graph convolutional networks use heterogeneous interaction information between users and items but lack sufficient use of homogeneous interaction information in the user and item graphs, thus it leads to the degradation of recommendation accuracy performance. For this purpose, this paper proposes some methods for constructing homogeneous interaction graph models that can be combined with heterogeneous interaction graphs to fully aggregate the node similarity and edge link information in the graph so that node embedding representations based on graph data can be better learned through graph convolutional networks. Experimental results based on several recommendation datasets show that the proposed homogeneous interaction graph can help the recommendation model to better mine the potential feature information and reduce the prediction error of ratings.
论文类型:论文集
学科门类:工学
文献类型:C
是否译文:否
发表时间:2023-01-12
收录刊物:EI